Use VCE Exam Simulator to open VCE files
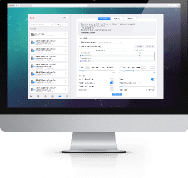
CSSBB ASQ Practice Test Questions and Exam Dumps
Question 1
Which type of control chart is primarily used to monitor the stability of the average value of a key metric over time?
A. NP Chart
B. Xbar-R Chart
C. I-MR Chart
D. C Chart
Correct Answer: B
Explanation:
Control charts are statistical tools used in quality control processes to determine whether a manufacturing or business process is in a state of control. Each type of control chart is designed for specific types of data and objectives, such as tracking averages, ranges, individual data points, or counts of defects. When the goal is to observe the stability of the average value of a process over time, one specific chart is particularly appropriate.
The Xbar-R Chart (B) is explicitly designed for tracking both the average (mean) and the range (variability) of a process using subgrouped data. The “Xbar” component monitors the mean value of measurements within each subgroup, and the “R” component monitors the range within each subgroup. This type of chart is best used when data is collected in small batches (usually 2–10 units per subgroup), allowing the user to observe both shifts in the average and changes in the consistency of the process.
Option A, NP Chart, is used to monitor the number of nonconforming units in a fixed-size sample. It is suitable for attribute data (pass/fail, defect/no defect), not for continuous data like measurement values. Therefore, it is not designed to track the average of a continuous metric.
Option C, I-MR Chart (Individuals and Moving Range Chart), is used when only individual measurements are available (i.e., sample size = 1), and subgroups cannot be formed. While it can monitor the central tendency (using the “I” chart) and variability (using the “MR” chart), it is generally considered a less sensitive tool compared to Xbar-R when subgroup data is available. The I-MR Chart is useful but not the best choice when subgroup data is an option.
Option D, C Chart, is used to monitor the number of defects (not defective items) in a sample, assuming the sample size remains constant. This is appropriate for count data rather than for analyzing the mean of continuous measurements.
To summarize, when the objective is to track the average value of a key process metric over time, especially with subgrouped continuous data, the Xbar-R Chart is the most appropriate and widely accepted tool in statistical process control. It provides the capability to detect both small and large shifts in the process mean, making it highly effective for ensuring the stability and consistency of processes.
Question 2
For her injection molding project, a Belt needed to monitor the percentage of defective units in a specific sample set.
What type of chart did she use to visualize this data?
A. Individual Chart
B. C Chart
C. Xbar Chart
D. P Chart
Answer: D
Explanation:
In Six Sigma and quality control processes, choosing the right type of control chart is essential for properly analyzing data and maintaining process stability. Different types of charts are designed for different kinds of data, such as variable data (measured on a continuous scale) or attribute data (counted in categories like defects or defectives). In this question, the focus is on tracking the percentage of defectives, which falls under attribute data.
A P Chart, or proportion chart, is specifically used to monitor the proportion of defective units in a process. It is ideal when you want to track the rate or percentage of nonconforming items in a given sample size. Each sample can have a different number of items, and the chart adjusts for that variability. This makes the P Chart the most suitable tool when the goal is to track and display the percentage of defectives over time.
Option A, Individual Chart, is used for continuous data when sample size is one. It plots individual data points over time to detect shifts or trends in the process mean. However, it is not suitable for percentage or attribute data because it assumes data are measured on a continuous scale, such as dimensions or weight—not pass/fail or defective categories.
Option B, C Chart, is used to track the number of defects per unit when the sample size remains constant. Importantly, it is not for tracking defectives (entire units that fail), but rather the number of defects within a unit, which can be multiple per item. Therefore, it is inappropriate for tracking proportions or percentages of defectives.
Option C, Xbar Chart, is designed for continuous data and is used to monitor the mean of a process based on samples of size greater than one. It assumes data is measurable in a continuous form (like time, weight, or volume), and is not meant for proportion or categorical data like defective vs. non-defective units.
In summary, since the Belt in the scenario is specifically tracking the percentage of defectives from a sample set—this is categorical attribute data best represented as proportions. A P Chart is the standard tool for this kind of analysis in Six Sigma and statistical process control. It allows for sample sizes to vary and provides a control limit structure that helps the user understand whether the variation in defectives is within expected statistical bounds.
Therefore, the correct answer is D.
Question 3
Which of the following charts can display conditions that would justify activating an Out-of-Control Action Plan (OCAP) for a process?
A. Xbar Chart
B. Time Series Chart
C. Neither
D. Both
Correct Answer: A
Explanation:
An Out-of-Control Action Plan (OCAP) is a predefined response or set of actions that should be taken when a process exhibits signs that it is no longer statistically in control. The ability to detect such signals depends on the type of chart used to monitor the process.
Option A, the Xbar Chart, is one of the primary tools used in statistical process control (SPC) for monitoring the average (mean) of a process over time. It is especially effective when used alongside a range chart (Xbar-R) or standard deviation chart (Xbar-S) for subgrouped data. The Xbar Chart provides statistically defined control limits, which make it possible to identify specific out-of-control signals, such as:
A point falling outside of the upper or lower control limits.
A run of several points all above or below the centerline.
Trends or cycles indicating a non-random pattern.
When such patterns occur, they signal that the process may be influenced by special causes rather than normal variation, thereby triggering the need for an OCAP. Therefore, an Xbar Chart clearly demonstrates the kinds of control violations that would justify initiating such a plan.
Option B, the Time Series Chart, displays data over time but does not inherently include statistically calculated control limits. This means while it shows trends and patterns, it does not provide a clear framework for determining whether the variation observed is within acceptable statistical boundaries. Without control limits or SPC rules applied, a time series chart alone cannot be reliably used to justify activating an OCAP.
Option C, “Neither,” is incorrect because the Xbar Chart alone is sufficient to meet the criteria for triggering OCAP under SPC rules.
Option D, “Both,” is incorrect because although a Time Series Chart offers a visual history of data, it lacks the statistical rigor necessary to support OCAP decisions unless augmented with control limits, at which point it effectively becomes a control chart like the Xbar Chart.
In conclusion, an Xbar Chart provides the statistical evidence needed to detect out-of-control conditions, thanks to its use of control limits and well-established rules for identifying abnormal patterns. It enables teams to act decisively through an OCAP by offering a clear indication when the process mean has shifted or when there are other non-random signals. A Time Series Chart, while useful for visualization, lacks the statistical criteria needed to justify such actions.
Question 4
Dr. Shewhart developed Control Charts to monitor process data over time. What features do these charts use to identify special cause variation?
A. Data shift analysis
B. Outlier analysis methods
C. Center Line and Control Limits
D. None of the above
Answer: C
Explanation:
Control Charts, also known as Shewhart Charts or Process Behavior Charts, are fundamental tools in statistical process control (SPC). They were introduced by Dr. Walter A. Shewhart in the 1920s while working at Bell Laboratories. The primary purpose of these charts is to determine whether a process is in statistical control—meaning the process variation is stable and predictable—or if there is some unusual or special cause variation that needs investigation.
At the heart of the Control Chart are three key components: the Center Line, the Upper Control Limit (UCL), and the Lower Control Limit (LCL). These components are calculated based on statistical principles and are derived from historical process data. They help distinguish between common cause variation, which is inherent in the process, and special cause variation, which is the result of external, unpredictable influences.
The Center Line typically represents the process average or mean. The Control Limits are calculated by taking the standard deviation of the process and adding or subtracting it (typically three standard deviations) to determine the UCL and LCL. When data points fall within the control limits and show no unusual patterns, the process is considered stable and in control.
To detect special cause variation, control charts rely on identifying:
Data points outside the UCL or LCL
Non-random patterns such as trends, cycles, or repeated sequences
Clusters of points on one side of the center line
Runs or sudden shifts in data over time
These patterns are not consistent with the expected random variation and indicate that something unusual is affecting the process, prompting further investigation.
Option A, data shift analysis, refers to a method of detecting changes in process averages over time but is not a formal feature built into Shewhart's control charts. It may be part of broader process analysis but not inherent in the structure of the chart.
Option B, outlier analysis methods, involves statistical techniques used to identify individual data points that are significantly different from the rest of the data. While control charts will flag extreme values, they do so within the context of control limits rather than generic outlier tests.
Option D, none of the above, is incorrect because Control Charts specifically use center lines and control limits to perform their primary function of identifying process variation.
In conclusion, the most accurate and complete answer is C. Control charts detect special cause variation by plotting data over time relative to a center line and statistically determined control limits, which was Shewhart’s foundational contribution to quality control and modern process improvement.
Question 5
Statistical Process Control focuses on two types of variation: Common Cause and which other type of variation?
A. Uncommon
B. Ordinary
C. Special
D. Selective
Correct Answer : C
Explanation:
Statistical Process Control (SPC) is a methodology used to monitor, control, and improve processes through statistical analysis. It focuses on identifying and managing the types of variation that occur within a process. These variations are generally categorized into two major types: Common Cause Variation and Special Cause Variation.
Common Cause Variation refers to the natural, inherent fluctuations that occur within a process over time. These are considered to be part of the system itself and result from the combined effect of many small, random factors. This type of variation is predictable within a certain range and does not indicate any fundamental issue with the process. Processes that only exhibit common cause variation are said to be in statistical control.
On the other hand, Special Cause Variation (Option C) refers to variation that arises from specific, identifiable sources that are not part of the regular process. These could include equipment malfunctions, operator errors, raw material defects, or sudden environmental changes. Special causes are not predictable, and their presence usually indicates that the process has gone out of control. When such variation is detected, it typically triggers investigation and corrective action.
The term “special cause” was introduced by Dr. W. Edwards Deming and is a foundational concept in quality management. It represents an indication that something outside the normal system is affecting the process, and therefore, requires immediate attention.
Option A, “Uncommon,” is a plausible-sounding term but is not the correct technical terminology used in SPC. It may imply something rare, but it does not capture the specific, identifiable nature of the causes being discussed.
Option B, “Ordinary,” is essentially a synonym for “common,” and therefore does not represent the contrasting category of variation that SPC aims to identify.
Option D, “Selective,” is not a term used in the context of statistical variation in process control. It might suggest some kind of intentional variation, which is not what SPC is concerned with when discussing types of variation.
In summary, SPC centers on distinguishing between common cause variation, which is built into the process, and special cause variation, which signals abnormal conditions or specific disruptions. Identifying and eliminating special causes is key to improving process stability and quality, while understanding common causes helps organizations recognize the limitations of their current systems and seek long-term improvements.
Question 6
Special Cause Variation can be divided into which two main categories?
A. Natural & Unnatural
B. Short Term & Long Term
C. Assignable & Pattern
D. Attribute & Discreet
Answer: C
Explanation:
In the context of statistical process control and quality management, variation in a process is typically classified into two broad types: common cause variation and special cause variation. Common cause variation, also referred to as natural or inherent variation, represents the expected and predictable fluctuation within a stable process. Special cause variation, on the other hand, occurs due to unexpected events or influences that are not part of the process’s normal behavior.
When examining special cause variation, it is often further divided into two conceptual subcategories: assignable and pattern-based. These help practitioners identify the nature of the variation and develop strategies for responding to it.
Assignable variation refers to changes that can be traced to a specific, identifiable source. These causes are often external or due to a sudden change in the process. For example, a machine malfunction, a raw material change, operator error, or environmental fluctuation could all cause assignable variation. The key here is that the variation has a clear origin that can be located and potentially eliminated or controlled.
Pattern variation, in contrast, refers to recurring behaviors or trends in the process data that indicate a non-random issue. Patterns might not be caused by a single assignable event but instead arise from systemic problems such as poor procedures, inadequate training, or long-term drift in equipment performance. These patterns are often visible in control charts through non-random sequences like trends, cycles, or shifts in the process mean.
Option A, natural and unnatural, is not a standard categorization. While “unnatural” might intuitively refer to special cause variation, the term “natural” usually applies to common cause variation, and these terms are not typically used as the formal subcategories of special cause variation.
Option B, short term and long term, describes time scales of variation but not categories of causes. Both short-term and long-term variations could arise from either common or special causes, so this distinction does not accurately reflect the classification of special causes.
Option D, attribute and discreet, refers to types of data, not types of variation. Attribute data refers to categorical data (like pass/fail), while discrete data typically includes countable items. These are unrelated to the concept of special cause variation itself.
Therefore, the most accurate classification for special cause variation is assignable and pattern, making C the correct answer. This distinction helps quality professionals understand whether a variation is due to a one-time event or an ongoing systemic issue, guiding the appropriate response to stabilize and improve the process.
Question 7
Range Charts are used to detect special causes occurring within subgroups of which type of chart?
A. Histograms
B. SPC Charts
C. NP Charts
D. Pareto Charts
Correct Answer : B
Explanation:
In the context of quality control and process monitoring, Range Charts (commonly referred to as R Charts) are a specific type of control chart used to track the dispersion or variability within subgroups over time. The primary purpose of an R Chart is to determine whether the amount of variation within a process is stable and consistent, or if it is being influenced by special causes that require investigation and corrective action.
Range Charts are most often used in conjunction with Xbar Charts as part of a system known as the Xbar-R Chart, which falls under the broader category of SPC Charts—Statistical Process Control Charts. These SPC Charts are the backbone of process quality monitoring in both manufacturing and service industries.
The Xbar Chart monitors the central tendency (average) of a process, while the R Chart monitors the range or spread within each subgroup. If the range of values within subgroups suddenly increases or decreases beyond expected statistical control limits, it indicates that special cause variation might be affecting the consistency of the process, even if the average remains within acceptable limits.
Option B, SPC Charts, is the correct answer because Range Charts are a specific type of SPC tool. They are designed to detect whether a process is statistically in control based on the variability within subgroups, which is central to identifying special causes.
Option A, Histograms, are useful for visualizing the overall distribution of data, but they do not show variation over time or help in identifying special causes within subgroups.
Option C, NP Charts, are used for attribute data to track the number of nonconforming units in fixed-size samples. They are not designed to monitor continuous data or subgroup variability and do not use range as a parameter.
Option D, Pareto Charts, are bar charts that prioritize problems or causes based on frequency or impact. They are useful for identifying the most significant sources of defects or failures but do not track process variation within subgroups over time.
To conclude, Range Charts are a core component of SPC Charts and are specifically used to detect abnormal variability within subgroups, which could indicate the presence of special causes. This ability to differentiate between normal process variation and unusual events is essential for maintaining quality and making informed process improvements.
Question 8
When monitoring a high-volume production process involving four machines, where both the average and variability of variable data need to be tracked,
Which SPC chart is the most appropriate choice?
A. Xbar-R Chart
B. Individual-MR Chart
C. NP Chart
D. CUSUM Chart
Answer: A
Explanation:
In statistical process control (SPC), selecting the correct control chart depends on several factors: the type of data (attribute or variable), the sample size, and the process characteristics being measured (such as mean, variability, or frequency). In this question, the focus is on variable data in a high-volume production setting involving multiple machines. Furthermore, both the mean (central tendency) and variability (spread) of the process are of interest. These clues point to a specific charting method that can handle subgroup data effectively.
The Xbar-R Chart is the best fit for this scenario. This type of control chart is specifically designed to monitor the average (X-bar) and the range (R) of a process when the data are collected in subgroups. It is commonly used when you are taking a small number of observations (typically 2 to 10) at regular intervals from each machine or process line.
In high-volume production environments, it’s standard practice to sample a few units regularly rather than measuring every unit. The Xbar-R chart allows you to keep track of the process average across these subgroups (Xbar Chart) and the amount of variation within the subgroups (Range Chart). This dual monitoring helps detect shifts in the process center and increases in process variation.
Option B, the Individual-MR (Moving Range) Chart, is used when only a single data point is collected per time period. It is ideal for low-volume or custom production where subgroup sampling isn't feasible. Since the scenario here involves high-volume production and multiple machines, more than one sample per time period would likely be collected, making the Individual-MR Chart less suitable.
Option C, the NP Chart, is used for attribute data when tracking the number of defectives in a fixed sample size. Since the question clearly states that the characteristic being measured is variable data (like weight, temperature, or length), the NP Chart is not applicable.
Option D, the CUSUM Chart, or Cumulative Sum Control Chart, is useful for detecting small shifts in the process mean over time. However, it’s more sophisticated and often used when subtle changes are important in a stable process. While useful in some contexts, it’s not the most straightforward or common first choice for routine high-volume production with multiple machines.
To summarize, in a setting with variable data, high production volume, multiple machines, and a need to monitor both mean and variability, the Xbar-R Chart provides a balanced and effective solution. It enables timely detection of both process drift and variability increases, allowing for corrective actions before defects escalate.
Thus, the correct answer is A.
Question 9
If a Belt completely removes a defect from the process using Poka-Yoke, should she still use a strong SPC system to monitor the relevant characteristic as an early warning?
A. True
B. False
Correct Answer : A
Explanation:
Poka-Yoke, a Japanese term meaning “mistake-proofing,” refers to any mechanism or procedure that helps an operator avoid (or prevent) mistakes in a process. When applied effectively, Poka-Yoke can eliminate specific types of defects entirely by ensuring that errors cannot occur in the first place—for example, through fixtures, sensors, or design changes that prevent incorrect assembly.
However, even when a defect is believed to be fully eliminated, it is still essential to maintain vigilant oversight. This is where Statistical Process Control (SPC) becomes important. SPC is not just a tool for tracking defects; it is primarily used to monitor variation in a process, and variation can signal deeper issues—such as wear-and-tear in machinery, operator inconsistencies, or environmental factors—that could reintroduce the potential for defects under certain conditions.
The statement in this question asserts that even after a Poka-Yoke solution removes a defect, a Belt should continue to monitor the relevant characteristic with a robust SPC system. This is absolutely correct and reflects best practices in Lean Six Sigma and quality management systems. Here's why:
Poka-Yoke is not always foolproof: While the intent is to prevent mistakes, physical devices or control systems can fail, degrade over time, or be bypassed by users. Continuous monitoring with SPC helps catch such lapses early.
SPC acts as an early warning system: Even if the defect does not reappear, variation in the process characteristics can indicate that the process is drifting or becoming unstable. Early detection allows proactive maintenance or process adjustments before quality issues arise.
Data-driven decision-making: Robust SPC systems provide historical data and trend analysis, which help identify patterns that may not be immediately obvious. This data supports ongoing process improvement efforts.
Regulatory and compliance standards: In many industries, ongoing monitoring is required to meet quality and compliance standards, even when Poka-Yoke solutions are in place.
Option A, True, is therefore the correct answer because it reflects the principle that SPC and Poka-Yoke complement each other. Poka-Yoke prevents mistakes, while SPC ensures ongoing process health and provides a safety net in case the Poka-Yoke mechanism fails or is compromised.
Option B, False, is incorrect because it assumes that Poka-Yoke alone is sufficient to guarantee process quality, which contradicts the layered defense philosophy advocated in quality management.
In summary, even after a process has been mistake-proofed through Poka-Yoke, continuing to monitor the process using SPC is essential to maintain confidence in the process stability and to detect any emerging issues.
Question 10
After completing a Lean Six Sigma project, the Belt prepares a Control Plan. What additional document does he create to ensure that the team knows how to respond if key performance metrics go out of specification?
A. Response Plan
B. Call List
C. Chain-of-Command
D. Defect Analysis Plan
Answer: A
Explanation:
In Lean Six Sigma (LSS), the final phase of a project is the Control phase. This stage focuses on ensuring that the gains made during the project are sustained over time. One of the key deliverables in this phase is the Control Plan, which serves as a roadmap for monitoring and maintaining the improved process. It typically includes what metrics are being tracked, how frequently they are measured, the tools used for monitoring, acceptable limits, and who is responsible for each task.
However, having a Control Plan alone is not sufficient. To handle unexpected deviations or situations where critical metrics move out of specification, the Belt must also create a Response Plan. This document outlines specific actions that team members must take when they detect a process that is drifting out of control. It acts as a contingency plan and ensures consistency in how issues are addressed, minimizing downtime or damage and promoting a proactive approach to process control.
A well-structured Response Plan generally includes:
Clear identification of which metrics, if deviated, require action
Defined thresholds that trigger a response
Step-by-step instructions on what actions to take
Roles and responsibilities for executing those actions
Contact information for escalation if necessary
Timeline expectations for each action step
This ensures that everyone involved in the process understands how to react swiftly and effectively to prevent defects from reaching customers and to bring the process back into control.
Option B, Call List, may be part of the Response Plan but is too narrow. It only refers to who to contact, not what actions to take.
Option C, Chain-of-Command, outlines reporting structures or authority levels but doesn't detail what actions to take when a process goes out of spec.
Option D, Defect Analysis Plan, is a more reactive document focused on understanding defects after they occur, rather than managing the process in real time to prevent them.
Therefore, to supplement the Control Plan and ensure a rapid, standardized response to deviations, the most appropriate and complete document is the Response Plan.
The correct answer is A.
Top Training Courses
SPECIAL OFFER: GET 10% OFF
This is ONE TIME OFFER
A confirmation link will be sent to this email address to verify your login. *We value your privacy. We will not rent or sell your email address.
Download Free Demo of VCE Exam Simulator
Experience Avanset VCE Exam Simulator for yourself.
Simply submit your e-mail address below to get started with our interactive software demo of your free trial.