Use VCE Exam Simulator to open VCE files
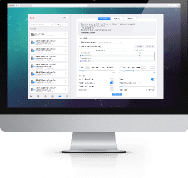
DP-600 Microsoft Practice Test Questions and Exam Dumps
You need to ensure that Contoso can use version control to meet the data analytics and general requirements. What should you do?
A. Store the semantic models and reports in Data Lake Gen2 storage.
B. Modify the settings of the Research workspaces to use a GitHub repository.
C. Modify the settings of the Research division workspaces to use an Azure Repos repository.
D. Store all the semantic models and reports in Microsoft OneDrive.
C. Modify the settings of the Research division workspaces to use an Azure Repos repository.
To fulfill Contoso's data analytics and general requirements, version control for semantic models and reports is a critical factor, especially for the Research division. Here's why option C is the best solution:
Azure Repos and Version Control: The requirement to use version control with support for branching is best achieved through Azure Repos. Azure Repos integrates well with Microsoft’s environment, allowing for robust version control and team collaboration, which meets the needs of Contoso's Research division's semantic models. It enables structured management of changes and provides an efficient workflow for maintaining multiple versions of semantic models, reports, and associated configurations. Additionally, Azure Repos can integrate directly with Fabric, making it ideal for this scenario.
Compatibility with Fabric: Fabric enables seamless integration with Azure Repos, which allows for managing the Research division’s workspaces and ensuring that models and reports are correctly versioned, supporting branching and collaboration among teams. This minimizes maintenance overhead and aligns with Contoso's general requirement to minimize implementation effort.
Minimizing Maintenance Effort: Azure Repos is an industry-standard tool for source control and fits seamlessly into the existing infrastructure for the Research division’s Fabric workspaces. It simplifies the development lifecycle by providing integrated support for version control without requiring external tools or additional configuration, which would have been more complicated with other options such as GitHub or OneDrive.
Exclusion of Other Options:
Option A (Data Lake Gen2 storage): While Data Lake Gen2 can store large datasets, it does not inherently provide version control or branching support, making it unsuitable for this requirement.
Option B (GitHub repository): GitHub is also a version control tool but is more oriented towards code repositories and might not integrate as smoothly with the Microsoft ecosystem, particularly Fabric and Power BI, compared to Azure Repos.
Option D (OneDrive): OneDrive is primarily a cloud storage service for file management, not suited for source control or versioning of semantic models and reports, making it unsuitable for this scenario.
Thus, Azure Repos provides the most effective and integrated solution for version control in this case.
Question No 2:
Group the Research division workspaces to enable department-based filtering in the OneLake data hub, with minimal effort and following the principle of least privilege.
A. Assign a department metadata tag to each workspace
B. Create a Fabric domain named "Research"
C. Add all workspaces to a single workspace collection
D. Assign a custom security group to each workspace
Microsoft Fabric uses domains as a logical grouping mechanism that enables better organization, discoverability, and access control across workspaces and assets in the OneLake data hub. A domain in Fabric can represent a business unit such as "Sales" or "Research" and is particularly useful when you want to filter or manage data assets by department or function.
By creating a Fabric domain named "Research" and assigning the Productline1ws and Productline2ws workspaces to this domain, Contoso can ensure that all related assets are logically grouped and easily discoverable via the OneLake interface. This approach meets the requirement for department-based filtering without needing to implement custom tagging or complex workspace collections.
Other options are less effective or more complex:
Metadata tags (Option A) can help with discovery but do not natively enable filtering in the OneLake data hub UI.
Workspace collections (Option C) are not a current feature in Fabric for this purpose.
Security groups (Option D) manage access, not logical grouping or filtering.
Using Fabric domains aligns with the principle of least privilege by allowing domain-level access controls and minimizes maintenance since grouping and filtering can be handled via Fabric’s built-in domain capabilities. This makes it the most efficient and scalable approach for managing department-level workspaces in a unified data platform like Microsoft Fabric.
Question No 3:
You need to recommend a solution to group the Research division workspaces according to Contoso’s requirements.
What should you include in the recommendation?
To answer, select the appropriate options in the answer area. NOTE: Each correct selection is worth one point.
Recommendation:
Use Fabric Workspaces: To effectively manage the workspaces, they should be logically grouped under Fabric Workspaces that match the product line structure for the Research division. This ensures seamless data management and supports the required analytics features.
Support OneLake Data Hub Filtering: Group the Research workspaces logically to facilitate filtering based on the department name. This enables efficient access and data management by product line and department, and ensures compliance with the filtering requirements.
Version Control Integration: Configure version control integration within the workspaces to ensure that all semantic models and reports for the Research division use version control that supports branching. This can be achieved through tools like Azure Repos, which will be used for versioning the models, ensuring consistency and maintaining a structured versioning process.
Use On-demand Capacity: For optimal performance and cost management, the Research division workspaces should leverage on-demand capacity with per-minute billing. This provides scalability and cost-effective resource allocation, as required by Contoso.
To meet the business needs, Contoso must ensure that all Research division workspaces are properly configured to handle the unique requirements of data management, version control, and data analytics.
The use of Fabric Workspaces to group workspaces based on product lines is crucial for the Research division’s needs. Each product line workspace should be isolated but still accessible under the same OneLake data hub for filtering purposes. By grouping the workspaces by department (Research division), the data filtering process will be more efficient, aligning with Contoso’s requirement for logical grouping.
Version control is a central aspect of the project, particularly for the semantic models and reports in the Research division. Using Azure Repos or another suitable version control tool ensures that semantic models are tracked, versions are controlled, and team collaboration is streamlined. This supports branching and allows for controlled changes and rollbacks when needed.
Additionally, enabling on-demand capacity ensures that the Research division only pays for the compute resources they use, aligning with the per-minute billing requirement. This also ensures cost-efficiency while providing the necessary computing power for complex analytics tasks.
In conclusion, grouping the Research workspaces logically, integrating version control, and using on-demand capacity are key steps to fulfilling Contoso’s detailed business, analytics, and data management requirements.
Contoso, Ltd. is working on refreshing the Orders table in the Online Sales department. The refresh process must minimize the number of rows added during the refresh, while meeting the semantic model requirements.
What should be included in the solution?
A. An Azure Data Factory pipeline that executes a Stored procedure activity to retrieve the maximum value of the OrderID column in the destination lakehouse.
B. An Azure Data Factory pipeline that executes a Stored procedure activity to retrieve the minimum value of the OrderID column in the destination lakehouse.
C. An Azure Data Factory pipeline that executes a dataflow to retrieve the minimum value of the OrderID column in the destination lakehouse.
D. An Azure Data Factory pipeline that executes a dataflow to retrieve the maximum value of the OrderID column in the destination lakehouse.
Answer:
A. An Azure Data Factory pipeline that executes a Stored procedure activity to retrieve the maximum value of the OrderID column in the destination lakehouse.
Explanation:
Contoso, Ltd. requires a solution for refreshing the Orders table while minimizing the number of rows added during the refresh. To achieve this, the most efficient method is to use a Stored Procedure activity in an Azure Data Factory (ADF) pipeline that retrieves the maximum value of the OrderID column in the destination lakehouse. This solution focuses on efficiently identifying the last processed order (i.e., the maximum OrderID) and updating only the subsequent new orders.
Here’s why Option A is the best approach:
Minimizing Added Rows: Since the OrderID is the sequence identifier for orders, querying for the maximum value allows you to identify the most recent order that has been processed in the lakehouse. You can then add only the new orders with an OrderID greater than the current maximum, minimizing the rows added during the refresh.
Efficient Data Loading: By retrieving the maximum OrderID, you ensure that the refresh operation pulls only the relevant data, avoiding the need to reprocess all rows in the Orders table. This method ensures that you only fetch new orders since the last update, which aligns with the semantic model requirements of minimizing data refresh.
Let’s examine why the other options are less suitable:
Option B: Using the minimum value of the OrderID is not ideal because it would mean selecting the oldest order or the first data point, which doesn’t address the need for efficient incremental updates (i.e., only pulling new data).
Option C and Option D: Both dataflows are designed to perform more complex transformations on data. However, in this case, executing a dataflow is less efficient than using a stored procedure to simply retrieve the maximum or minimum value from the table.
Therefore, Option A—using an Azure Data Factory pipeline with a Stored Procedure that retrieves the maximum OrderID—is the most efficient and appropriate method for minimizing the rows added during the refresh process. This solution ensures a fast and optimized data update workflow.
A.spark.read.format(“delta”).load(“Tables/productline1/ResearchProduct”)
B. spark.sql(“SELECT * FROM Lakehouse1.ResearchProduct”)
C. external_table(‘Tables/ResearchProduct’)
D. external_table(ResearchProduct)
Answer:
A. spark.read.format(“delta”).load(“Tables/productline1/ResearchProduct”)
Explanation:
Contoso, Ltd. uses Lakehouse1 to store Productline1 data for the Research division. To access the data in Fabric notebooks, the appropriate syntax must be used to load the data, which is stored in Delta format.
Option A is the correct choice because it specifies the use of the Delta format for reading data, which is the format used by Lakehouse1 to store data for the Research division. In this case, Delta is the preferred storage format due to its ability to handle ACID transactions and optimize query performance, especially for big data scenarios in cloud-based environments like Fabric.
Here’s why Option A is the correct choice:
Delta Format: The syntax spark.read.format(“delta”) indicates that the data is stored in the Delta Lake format, which is commonly used in modern data lakes for its support for ACID transactions and time travel capabilities.
Loading Data: The load() function is used to load the data from the specified location, in this case, the ResearchProduct shortcut that links to the data stored in Lakehouse1.
Now, let’s consider the other options:
Option B uses SQL syntax, but since we are working within a Fabric notebook, which uses Spark, the correct approach is to use Spark's built-in reading functions like spark.read.format(), rather than a SQL query.
Options C and D are referring to the use of external tables, which are more typically used for registering and querying external data sources, not for directly reading data from a Lakehouse in a Fabric notebook.
Thus, Option A is the correct syntax for accessing the Research division's data for Productline1 in Lakehouse1 within a Fabric notebook.
Litware, Inc. is a manufacturing company that has a presence throughout North America. The analytics team at Litware is composed of data engineers, analytics engineers, data analysts, and data scientists. The company is planning to enable Fabric features within its existing Microsoft Power BI tenant, and the team aims to create a proof of concept (PoC) to test the functionality of Fabric for its data store, semantic models, and reports.
Litware's existing data environment includes Product data, Customer Satisfaction data (comprising Survey, Question, and Response tables), and a variety of issues involving large volumes of semi-structured data.
Litware plans to enable Fabric features in its existing tenant, with the goal of creating a new data store for a PoC. This store will support the loading and transformation of data from OneLake, with various workspaces, including AnalyticsPOC, DataEngPOC, and DataSciPOC.
In the AnalyticsPOC workspace, the data store will be created, alongside semantic models, interactive reports, dataflows, and notebooks. Additionally, data engineers will load data into OneLake, and data engineers and analytics engineers will work with the data to cleanse, merge, and transform it into a dimensional model.
The dimensional model will include a date dimension (from 2010 to the current year), and the product pricing group logic will be maintained as follows:
Low pricing: List price ≤ 50
Medium pricing: 50 < List price ≤ 1,000
High pricing: List price > 1,000
The security model will enforce least privilege by granting different levels of access to various teams, ensuring that Fabric administrators and specific roles (e.g., data engineers, data analysts) can interact with the data store according to their responsibilities.
You are tasked with resolving an issue related to pricing group classification in the T-SQL statement. Specifically, you need to complete the T-SQL statement that implements the pricing logic for product data. You must ensure that the logic correctly classifies product prices into Low, Medium, and High categories.
How should you complete the T-SQL statement? To answer, select the appropriate options in the answer area. NOTE: Each correct selection is worth one point.
In this question, Litware needs a T-SQL statement that correctly classifies product prices into three pricing groups: Low, Medium, and High. This is a common scenario in data processing and involves using a CASE statement in SQL, which allows conditional logic to be applied based on specific criteria.
The T-SQL CASE statement works by evaluating a series of conditions in order:
The first condition checks if the ListPrice is less than or equal to 50. If this condition is true, the product is classified as Low.
The second condition checks if the ListPrice is greater than 50 and less than or equal to 1,000. If this condition holds true, the product is classified as Medium.
The third condition checks if the ListPrice is greater than 1,000. If this condition is satisfied, the product is classified as High.
This logical structure ensures that the pricing group classification is consistent with the rules provided in the requirements and resolves the issue with inconsistent classifications across the various systems and models.
By completing the statement as shown, the pricing logic is applied correctly in the data store and semantic models, ensuring consistency for all reporting and analytics workflows. The use of T-SQL ensures that the data can be queried efficiently and that the classification logic is applied automatically during data ingestion or querying. This meets the product pricing group requirement and supports Litware’s goals for creating a seamless data pipeline and report generation.
In the case study provided for Litware, Inc., the company plans to enable Fabric features and create a proof of concept (PoC) for its analytics team. They aim to develop a data store, implement semantic models, and create interactive reports using data from a variety of sources. The data store must support T-SQL or Python access, semi-structured and unstructured data, row-level security (RLS), and data transformation processes. Given these requirements, which type of data store should be recommended in the AnalyticsPOC workspace?
A. A data lake
B. A warehouse
C. A lakehouse
D. An external Hive metastore
Litware, Inc. has several key requirements for their proof of concept (PoC) project in the AnalyticsPOC workspace. These requirements provide insights into the ideal type of data store that should be recommended. Let’s break down the considerations and why a lakehouse is the best choice:
Data Types and Integration: Litware needs a data store that can handle both structured and semi-structured/unstructured data. A lakehouse combines the best features of a data lake (which can handle raw, semi-structured, and unstructured data) and a data warehouse (which is optimized for structured data with query performance and analytics). A lakehouse provides a unified platform for storing and processing all types of data, making it an ideal choice for Litware's diverse data requirements.
T-SQL and Python Access: The data store needs to support T-SQL or Python access for querying the data. Lakehouses are capable of supporting both SQL-based querying (as in data warehouses) and Python-based operations, typically through frameworks such as Apache Spark, making it easy for analysts, engineers, and data scientists to work with the data.
Row-Level Security (RLS): Implementing RLS on a lakehouse is feasible, providing control over who can access specific data based on user roles, which aligns with Litware’s security requirements. A lakehouse also allows granular data access, such as ensuring data analysts only see certain objects and enforcing the principle of least privilege.
Data Transformation and Processing: Litware’s analytics team will need to cleanse, merge, and transform data. Lakehouses support robust data transformation processes (through tools like Delta Lake or Apache Spark), allowing for efficient ETL (Extract, Transform, Load) workflows.
Cost Efficiency and Scalability: A lakehouse enables scalable storage, supporting the volume of data that Litware needs to manage. As Litware plans to use the proof of concept (PoC) with trial Fabric capacities, a lakehouse can also be a cost-effective solution because it allows storing large volumes of raw data before transforming it into a structured format.
In conclusion, a lakehouse fits the needs of Litware’s data engineering, data analysis, and security requirements, making it the best recommendation for their PoC data store in the AnalyticsPOC workspace.
Top Training Courses
SPECIAL OFFER: GET 10% OFF
This is ONE TIME OFFER
A confirmation link will be sent to this email address to verify your login. *We value your privacy. We will not rent or sell your email address.
Download Free Demo of VCE Exam Simulator
Experience Avanset VCE Exam Simulator for yourself.
Simply submit your e-mail address below to get started with our interactive software demo of your free trial.