Use VCE Exam Simulator to open VCE files
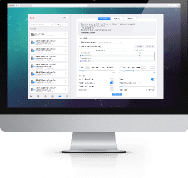
Professional Machine Learning Engineer Google Practice Test Questions and Exam Dumps
Question No 1:
You are building an ML model to detect anomalies in real-time sensor data. You will use Pub/Sub to handle incoming requests. You want to store the results for analytics and visualization. How should you configure the pipeline?
A. 1 = Dataflow, 2 = AI Platform, 3 = BigQuery
B. 1 = DataProc, 2 = AutoML, 3 = Cloud Bigtable
C. 1 = BigQuery, 2 = AutoML, 3 = Cloud Functions
D. 1 = BigQuery, 2 = AI Platform, 3 = Cloud Storage
Correct Answer: A
Explanation:
To design an effective pipeline for real-time anomaly detection in sensor data, using Google Cloud Platform (GCP) services, you must consider three key stages: stream ingestion and processing, machine learning model execution, and data storage for visualization and analytics.
Let’s break down the roles of each component described in option A, and why this configuration is the most appropriate:
1 = Dataflow
Dataflow is GCP’s fully managed service for stream and batch data processing, built on Apache Beam. It integrates natively with Pub/Sub for real-time data ingestion and processing. Since the sensor data is arriving via Pub/Sub, using Dataflow to process this stream in real time—cleaning it, extracting features, or transforming it—is ideal. It can also call external ML models or inference endpoints mid-stream, making it suitable for anomaly detection tasks
2 = AI Platform
AI Platform (now often integrated into Vertex AI) is used for training, deploying, and serving machine learning models. In this context, you would train your anomaly detection model on historical data and then deploy it as a prediction service. Dataflow can send batches or single-point features to the AI Platform's endpoint and receive anomaly scores in response. This allows for real-time inference within the pipeline.
3 = BigQuery
BigQuery is GCP’s highly scalable data warehouse optimized for analytics. After processing and scoring the sensor data, you would store the results in BigQuery for historical analysis, dashboarding (e.g., via Looker Studio or Tableau), and advanced querying. This setup supports rich visualizations and trend detection, which are essential for anomaly analysis over time
Now let’s examine why the other options are incorrect:
DataProc is a managed Hadoop/Spark service—more suitable for batch processing than real-time. AutoML is a good low-code ML tool but not ideal for real-time predictions. Cloud Bigtable is a NoSQL database suited for fast lookups, not analytics or visualization.
BigQuery as the first step is incorrect because it’s not designed to handle streaming data ingestion directly. AutoML is not ideal for real-time inference. Cloud Functions are good for lightweight triggers, but not scalable for continuous anomaly scoring in streams.
This setup is backward: BigQuery should not be the starting point. AI Platform is correct for ML inference, but Cloud Storage is more appropriate for unstructured object storage (e.g., logs, files), not for analytical querying or dashboarding.
In conclusion, the pipeline in option A—using Dataflow for stream processing, AI Platform for real-time ML inference, and BigQuery for storage and analytics—provides the most efficient, scalable, and analytics-ready solution for real-time anomaly detection in sensor data streams.
Question No 2:
Your organization wants to make its internal shuttle service route more efficient. The shuttles currently stop at all pick-up points across the city every 30 minutes between 7 am and 10 am.
The development team has already built an application on Google Kubernetes Engine that requires users to confirm their presence and shuttle station one day in advance. What approach should you take?
A. 1. Build a tree-based regression model that predicts how many passengers will be picked up at each shuttle station. 2. Dispatch an appropriately sized shuttle and provide the map with the required stops based on the prediction.
B. 1. Build a tree-based classification model that predicts whether the shuttle should pick up passengers at each shuttle station. 2. Dispatch an available shuttle and provide the map with the required stops based on the prediction.
C. 1. Define the optimal route as the shortest route that passes by all shuttle stations with confirmed attendance at the given time under capacity constraints. 2. Dispatch an appropriately sized shuttle and indicate the required stops on the map.
D. 1. Build a reinforcement learning model with tree-based classification models that predict the presence of passengers at shuttle stops as agents and a reward function around a distance-based metric. 2. Dispatch an appropriately sized shuttle and provide the map with the required stops based on the simulated outcome.
Answer: C
Explanation:
This scenario presents a route optimization problem constrained by real-time confirmed user attendance data. Since users confirm their pickup locations in advance via the application, the system already knows which stations will have passengers. Therefore, predictive modeling approaches such as regression (as in A) or classification (as in B) are unnecessary here. Similarly, more complex solutions like reinforcement learning (as in D) may be overkill and impractical for a task with deterministic inputs.
Let’s analyze each option:
This suggests predicting how many passengers will be picked up at each station. However, the system already requires passengers to confirm their station one day in advance. This means you know exactly how many passengers to expect at each station. There's no need to estimate or predict this number through a regression model. Adding machine learning here introduces unnecessary complexity.
Here, a classification model is proposed to predict whether the shuttle should pick up passengers at a stop. Again, this is redundant because the attendance data from the app already tells you this information explicitly. There's no ambiguity requiring a classifier to resolve. Using a classifier would be a misapplication of ML when deterministic data is already available.
Additionally, by matching the route with shuttle capacity, the solution remains scalable and practical for varying passenger loads. This solution also lends itself to known algorithms and solvers (e.g., Google OR-Tools), making implementation feasible and cost-effective.
Although interesting academically, this approach is unnecessarily complex. Reinforcement learning is ideal for environments with sequential decision-making under uncertainty. But in this case, the future state (passenger attendance) is already known and static. Implementing and tuning RL models here adds computational overhead with little gain in performance, especially when simpler optimization algorithms can solve the problem directly.
Conclusion:
Since confirmed attendance data is available in advance, there's no need for predictive models. The best solution is a deterministic optimization algorithm that calculates the shortest route to all confirmed stops, considering shuttle capacity. This is exactly what Option C proposes, making it the most logical, efficient, and technically sound solution.
Question No 3:
You were asked to investigate failures of a production line component based on sensor readings. After receiving the dataset, you discover that less than 1% of the readings are positive examples representing failure incidents. You have tried to train several classification models, but none of them converge.
How should you resolve the class imbalance problem?
A. Use the class distribution to generate 10% positive examples.
B. Use a convolutional neural network with max pooling and softmax activation.
C. Downsample the data with upweighting to create a sample with 10% positive examples.
D. Remove negative examples until the numbers of positive and negative examples are equal.
Correct Answer: C
Explanation:
The scenario describes a severe class imbalance problem, where the dataset has less than 1% positive examples, which represent failure incidents. This imbalance makes it difficult for most machine learning models to learn to recognize the minority class, as the model can simply predict the majority class (non-failure) and still achieve deceptively high accuracy.
Class imbalance is common in rare event prediction tasks such as fraud detection, machine failure, or disease diagnosis. In such cases, the challenge is to help the model give enough attention to the minority class so that it can learn meaningful patterns for identifying it. Here’s a breakdown of the choices and why C is correct:
C. Downsample the data with upweighting to create a sample with 10% positive examples
This is the correct and most balanced solution. It involves two actions:
Downsampling the majority class (negative examples) reduces the number of examples from the dominant class, helping to balance the dataset without eliminating the minority class.
Upweighting the positive examples ensures the model considers them more heavily during training, compensating for their scarcity in the full dataset.
Together, these techniques help your classifier learn features from both classes more effectively without overfitting to a drastically smaller dataset or ignoring valuable negative samples entirely. Creating a sample with 10% positive examples is a practical target to improve class balance while retaining training quality.
A. Use the class distribution to generate 10% positive examples
This option suggests generating synthetic positive examples (possibly through techniques like SMOTE or data augmentation), but it lacks clarity. Simply adjusting the distribution without stating how the new data is generated can result in overfitting, misrepresentation, or biased training. Additionally, generating 10% positives without meaningful synthesis might distort the feature space.
B. Use a convolutional neural network with max pooling and softmax activation
This refers to using CNNs, which are powerful for image or spatial data, not necessarily tabular sensor data. Also, the architectural changes (CNNs and softmax) do not address class imbalance. The model type alone does not resolve the issue of underrepresented failure cases.
D. Remove negative examples until the numbers of positive and negative examples are equal
While this method—random undersampling—can balance classes, it discards most of your data, especially valuable negative examples that could help the model distinguish between normal and abnormal patterns. If you have millions of examples, you risk significant loss of information and generalizability.
In summary, the best practice in this context is option C, where you combine downsampling the overwhelming negative class and upweighting the scarce positive examples to reach a more effective training distribution. This approach ensures model convergence and improves the learning of failure incident patterns without overly compromising data integrity.
Question No 4:
You want to rebuild your ML pipeline for structured data on Google Cloud. You are using PySpark to conduct data transformations at scale, but your pipelines are taking over 12 hours to run. To speed up development and pipeline run time, you want to use a serverless tool and SQL syntax. You have already moved your raw data into Cloud Storage.
How should you build the pipeline on Google Cloud while meeting the speed and processing requirements?
A. Use Data Fusion’s GUI to build the transformation pipelines, and then write the data into BigQuery.
B. Convert your PySpark into SparkSQL queries to transform the data, and then run your pipeline on Dataproc to write the data into BigQuery.
C. Ingest your data into Cloud SQL, convert your PySpark commands into SQL queries to transform the data, and then use federated queries from BigQuery for machine learning.
D. Ingest your data into BigQuery using BigQuery Load, convert your PySpark commands into BigQuery SQL queries to transform the data, and then write the transformations to a new table.
Answer: D
Explanation:
This question focuses on building a faster, scalable machine learning (ML) pipeline using structured data on Google Cloud, with a preference for serverless solutions and SQL-based transformations. Your current approach with PySpark is not performing well—taking more than 12 hours to execute—which indicates a need to optimize for speed and efficiency by switching to tools better suited for serverless and highly parallelized processing.
Let’s analyze each of the choices based on these goals:
Cloud Data Fusion is a managed ETL service that offers a graphical interface to build and orchestrate data pipelines. While it provides low-code/no-code development, it is not fully serverless and may not be as performant as BigQuery for large-scale SQL-based transformations. Furthermore, Data Fusion pipelines typically run on Dataproc (a Spark-based engine), which doesn't solve your current problem of long PySpark execution times. If the goal is to move away from Spark and toward SQL-based serverless processing, Data Fusion isn't the most efficient or relevant solution.
This option sticks with Dataproc, Google’s managed Spark and Hadoop service. While SparkSQL is an improvement over using native PySpark in terms of syntax for SQL-style transformations, Dataproc is not serverless. It still requires cluster management, incurs startup/teardown overhead, and typically can't match the performance and scaling benefits of BigQuery. Staying in the Spark ecosystem contradicts the requirement to reduce runtime and switch to serverless and SQL-based approaches.
Cloud SQL is Google’s managed relational database, supporting MySQL, PostgreSQL, and SQL Server. It's suitable for transactional databases, not large-scale analytical workloads. Moving raw structured data into Cloud SQL adds unnecessary overhead, storage limits, and performance constraints—especially if the data is already in Cloud Storage and can be ingested directly into BigQuery. Furthermore, federated queries from BigQuery into Cloud SQL may introduce latency and complexity, defeating the purpose of speeding up your pipeline.
This is the most efficient and scalable approach. Here’s why:
Serverless Architecture: BigQuery is a fully serverless, highly parallelized data warehouse designed for large-scale analytical queries and ML workloads.
Performance: BigQuery can process terabytes to petabytes of data quickly using a distributed architecture, significantly cutting down pipeline runtime compared to Spark-based processing.
Ease of Use: BigQuery supports standard SQL, which you can use to reimplement your PySpark logic into SQL queries. This aligns with your desire to switch to a SQL-based syntax.
Integration with ML: BigQuery integrates directly with BigQuery ML, allowing you to build and train ML models using SQL, and even export data to Vertex AI or other ML platforms.
Simplicity: Loading data directly from Cloud Storage using BigQuery Load jobs is efficient and doesn’t require intermediate steps through other services.
Cost Efficiency: Since BigQuery separates storage and compute, you only pay for the compute time used during query execution. There's no need to manage infrastructure or worry about idle resources.
Conclusion:
Option D meets all the outlined requirements: it's serverless, supports SQL syntax, dramatically improves performance and scalability, and removes the need for Spark-based tools like Dataproc or Data Fusion. By loading data directly into BigQuery, transforming it via BigQuery SQL, and materializing results into new tables, your ML pipeline becomes faster, more maintainable, and cost-efficient.
Question No 5:
You manage a team of data scientists who use a cloud-based backend system to submit training jobs. This system has become very difficult to administer, and you want to use a managed service instead.
The data scientists you work with use many different frameworks, including Keras, PyTorch, theano, Scikit-learn, and custom libraries. What should you do?
A. Use the AI Platform custom containers feature to receive training jobs using any framework.
B. Configure Kubeflow to run on Google Kubernetes Engine and receive training jobs through TF Job.
C. Create a library of VM images on Compute Engine, and publish these images on a centralized repository.
D. Set up Slurm workload manager to receive jobs that can be scheduled to run on your cloud infrastructure.
Answer : A
Explanation:
In this scenario, the key requirements are:
Use of a managed service to simplify administration.
Support for multiple machine learning frameworks, including custom libraries.
Flexibility and ease of job submission across different frameworks.
Let’s evaluate each option:
Option A: Use the AI Platform custom containers feature to receive training jobs using any framework.
This is the correct choice. AI Platform (now part of Vertex AI) supports custom containers, which allow you to define your training environment entirely, including the ML framework, Python packages, and any custom logic or dependencies. Since your team uses Keras, PyTorch, Theano, Scikit-learn, and other custom libraries, this option provides the flexibility you need without requiring you to build and maintain your own orchestration infrastructure. Furthermore, AI Platform (Vertex AI) is a managed service, so it relieves you from the burden of maintaining servers or orchestration engines.
By using custom containers, you can build a Docker image with all the required dependencies and push it to a container registry (e.g., Artifact Registry or Docker Hub). The AI Platform will then use that image to spin up a training environment, run your job, and shut down automatically, ensuring high scalability and efficient use of resources.
Option B: Configure Kubeflow to run on Google Kubernetes Engine and receive training jobs through TF Job.
While Kubeflow offers a powerful way to manage machine learning workflows, it is not a managed service. You would still be responsible for setting up and maintaining the Google Kubernetes Engine (GKE) cluster, which involves considerable administrative effort—something you're trying to avoid. Furthermore, TFJob is more tailored to TensorFlow, which may not work seamlessly with other frameworks like Theano or Scikit-learn. This option does not align well with the goal of reducing system administration overhead.
Option C: Create a library of VM images on Compute Engine, and publish these images on a centralized repository.
Although this provides flexibility in terms of custom environments, it does not provide a managed service experience. Your team would still need to manually manage the lifecycle of these VMs, handle job orchestration, and monitor workloads, which contradicts your goal to simplify administration.
Option D: Set up Slurm workload manager to receive jobs that can be scheduled to run on your cloud infrastructure.
Slurm is a popular open-source job scheduler, widely used in HPC environments. However, it requires substantial effort to set up and maintain, particularly in a cloud environment. This approach is far from being a managed service and introduces significant administrative complexity, making it a poor fit for your use case.
Conclusion:
Option A provides a fully managed solution that allows data scientists to use any ML framework, simplifies job submission, and reduces administrative burden. This makes it the most appropriate and scalable solution for your diverse team of data scientists.
Question No 6:
You work for an online retail company that is creating a visual search engine. You have set up an end-to-end ML pipeline on Google Cloud to classify whether an image contains your company's product. Expecting the release of new products in the near future, you configured a retraining functionality in the pipeline so that new data can be fed into your ML models.
You also want to use AI Platform's continuous evaluation service to ensure that the models have high accuracy on your test dataset. What should you do?
A. Keep the original test dataset unchanged even if newer products are incorporated into retraining.
B. Extend your test dataset with images of the newer products when they are introduced to retraining.
C. Replace your test dataset with images of the newer products when they are introduced to retraining.
D. Update your test dataset with images of the newer products when your evaluation metrics drop below a pre-decided threshold.
Correct Answer: B
Explanation:
In a production-grade machine learning (ML) system, especially one that evolves with new classes or product variants over time—as in this visual search engine—the test dataset must be representative of the model's current and future prediction scope. When using AI Platform’s continuous evaluation service, maintaining an up-to-date and representative test dataset is essential for meaningful performance metrics.
Here’s why B is the best choice:
This approach ensures that your test dataset evolves alongside your model. As new products are added to the model through retraining, it's critical to also include images of these products in your test dataset so that evaluation remains accurate and comprehensive. This method retains the integrity of previous testing (to monitor regressions) and includes new data to validate performance on new products. By extending the test dataset instead of replacing it, you maintain backward compatibility while expanding to cover new prediction targets.
In other words, your test set continues to test the model's ability to handle both existing and newly introduced products, which aligns with real-world use cases. This allows continuous evaluation to provide feedback on how well the model generalizes to the full product catalog.
A. Keep the original test dataset unchanged even if newer products are incorporated into retraining
This is a poor strategy because your evaluation will be blind to the new products. Your retrained model may make poor predictions on new classes or instances that aren't represented in the old test set, yet your evaluation metrics will remain artificially high. That’s misleading and undermines the whole point of continuous evaluation.
C. Replace your test dataset with images of the newer products when they are introduced to retraining
Completely replacing the test dataset causes loss of historical performance tracking. It makes it impossible to measure whether the model continues to perform well on older product classes. In production, you often need to guarantee backward compatibility—new models should continue to recognize older products.
D. Update your test dataset with images of the newer products when your evaluation metrics drop below a pre-decided threshold
This is reactive rather than proactive. It introduces lag in evaluation, as you're only updating the test set after degradation is detected—potentially too late for certain business-critical applications. Additionally, metrics might not drop immediately if newer classes are missing from evaluation, masking the problem.
Option B is the most robust and forward-thinking solution. It keeps your evaluation process comprehensive, fair, and aligned with the model’s scope. It ensures that AI Platform’s continuous evaluation accurately reflects the model's current capabilities and helps prevent silent failures on newly introduced products.
Question No 7:
You need to build classification workflows over several structured datasets currently stored in BigQuery. Because you will be performing the classification several times, you want to complete the following steps without writing code: exploratory data analysis, feature selection, model building, training, and hyperparameter tuning and serving.
What should you do?
A. Configure AutoML Tables to perform the classification task.
B. Run a BigQuery ML task to perform logistic regression for the classification.
C. Use AI Platform Notebooks to run the classification model with pandas library.
D. Use AI Platform to run the classification model job configured for hyperparameter tuning.
Answer: A
Explanation:
The scenario described involves working with structured datasets already in BigQuery and aims to repeatedly perform a classification task that includes exploratory data analysis (EDA), feature selection, model training, hyperparameter tuning, and serving—without writing any code. This last requirement eliminates any solution that depends on scripting in Python, SQL, or other programming languages.
Let’s review the options one by one to identify the best fit.
AutoML Tables is a code-free, fully managed, automated machine learning service offered by Google Cloud. It is specifically designed for structured data and is well-suited for business users or data analysts who prefer graphical interfaces or want to build ML models without having to code. Here’s how AutoML Tables supports each of the requirements:
Exploratory Data Analysis (EDA): AutoML Tables automatically provides summary statistics and distributions of features.
Feature Selection: The system automatically handles feature engineering and selection based on predictive power.
Model Building and Training: It supports various model architectures tailored for classification tasks and trains them using AutoML’s backend engine.
Hyperparameter Tuning: AutoML Tables performs hyperparameter tuning automatically during training to optimize performance.
Model Serving: Once trained, models can be easily deployed and served via a hosted endpoint without writing deployment scripts or managing infrastructure.
In addition, AutoML Tables integrates directly with BigQuery, allowing you to select datasets from BigQuery through the interface. This means no data movement or conversion is needed.Because AutoML Tables meets all of your stated requirements—including no-code development, repeated execution, and full model lifecycle support—Option A is the ideal choice.
BigQuery ML allows you to build and train ML models directly in SQL. While powerful and tightly integrated with BigQuery, BigQuery ML still requires writing SQL queries. It can support logistic regression and even some hyperparameter tuning, but it does not provide a full GUI-based workflow for EDA, feature engineering, or visual model evaluation. Because your requirement specifically says no code, this option doesn't meet the criteria.
This approach involves Python programming, likely using pandas, scikit-learn, or TensorFlow. While it's flexible and powerful, it requires writing code in a Jupyter environment, which goes directly against your requirement for a no-code solution. Also, AI Platform Notebooks do not provide out-of-the-box GUI tools for model building or training without scripting.
While AI Platform (now part of Vertex AI) does support powerful training and tuning workflows, it requires users to create and submit custom training jobs, usually written in Python or TensorFlow. This option provides a robust ML pipeline, but it’s not no-code. The user would have to configure and launch jobs programmatically or via command-line interfaces, which is beyond the requested scope of a no-code approach.
Conclusion:
Only AutoML Tables offers an end-to-end, no-code platform for building classification models on structured data, complete with EDA, feature selection, model building, training, hyperparameter tuning, and model serving, all through a graphical interface. The tight integration with BigQuery further strengthens its suitability for the scenario.
Thus, A is the correct answer.
Question No 8:
You work for a public transportation company and need to build a model to estimate delay times for multiple transportation routes. Predictions are served directly to users in an app in real time. Because different seasons and population increases impact the data relevance, you will retrain the model every month. You want to follow Google-recommended best practices.
How should you configure the end-to-end architecture of the predictive model?
A. Configure Kubeflow Pipelines to schedule your multi-step workflow from training to deploying your model.
B. Use a model trained and deployed on BigQuery ML, and trigger retraining with the scheduled query feature in BigQuery.
C. Write a Cloud Functions script that launches a training and deploying job on AI Platform that is triggered by Cloud Scheduler.
D. Use Cloud Composer to programmatically schedule a Dataflow job that executes the workflow from training to deploying your model.
Answer .A
Explanation:
This question centers around building an end-to-end, real-time machine learning system that includes regular retraining, model deployment, and low-latency prediction serving. Furthermore, the solution must align with Google Cloud’s recommended best practices, which emphasize automation, modularity, and scalability using managed ML workflows.
Let’s analyze each option:
Option A: Configure Kubeflow Pipelines to schedule your multi-step workflow from training to deploying your model.
This is the correct choice. Kubeflow Pipelines is a Google-recommended tool for managing end-to-end machine learning workflows, especially when retraining is periodic and the pipeline includes multiple steps such as data preprocessing, model training, evaluation, and deployment. Since your use case involves monthly retraining, this fits perfectly with Kubeflow’s ability to define reusable pipeline components and schedule retraining workflows. It also integrates well with Vertex AI, offering scalable training and managed deployment of models for real-time inference. This provides a structured, repeatable, and maintainable solution and supports Google’s best practices for production ML systems.
Option B: Use a model trained and deployed on BigQuery ML, and trigger retraining with the scheduled query feature in BigQuery.
BigQuery ML is excellent for SQL-based models and rapid prototyping where data already resides in BigQuery. However, it is not designed for complex, multi-step ML workflows or real-time serving to users. Its support for real-time inference is limited compared to managed ML endpoints like those provided by Vertex AI. Also, BigQuery ML doesn’t provide robust mechanisms for deploying models for live app integration or integrating custom logic in the training pipeline, which is needed in this scenario.
Option C: Write a Cloud Functions script that launches a training and deploying job on AI Platform that is triggered by Cloud Scheduler.
While this method is technically viable, it is less scalable and harder to manage as your ML pipeline grows. Hardcoding orchestration logic in Cloud Functions is not aligned with Google Cloud’s best practices for ML systems. There’s limited visibility, debugging, and maintainability with this custom scripting approach, especially when multiple steps are involved (like data preparation, validation, model evaluation, rollback, etc.). Also, it doesn't naturally support pipeline versioning and monitoring.
Option D: Use Cloud Composer to programmatically schedule a Dataflow job that executes the workflow from training to deploying your model.
Cloud Composer, based on Apache Airflow, is a strong tool for orchestration but is generally more suitable for ETL workflows and batch data pipelines, not for building and managing complex ML pipelines. While it is possible to use Cloud Composer to trigger training and deployment workflows, it lacks the tight integration with ML-specific components like model evaluation, metadata tracking, and artifact lineage that Kubeflow Pipelines provides out of the box. Also, using Dataflow for training/deploying models is unconventional and not the primary use case of the tool—it’s better suited for stream and batch data processing, not ML orchestration.
Conclusion:
Option A is the most appropriate and aligns with Google’s recommended architecture for managing repeatable ML workflows, especially when retraining is required regularly and inference must be available in real time. Kubeflow Pipelines on Vertex AI ensures scalability, reproducibility, and maintainability of the entire ML lifecycle from data ingestion to model deployment, while also supporting rich integrations and pipeline monitoring.
Question No 9:
You are developing ML models with AI Platform for image segmentation on CT scans. You frequently update your model architectures based on the newest available research papers, and have to rerun training on the same dataset to benchmark their performance.
You want to minimize computation costs and manual intervention while having version control for your code. What should you do?
A. Use Cloud Functions to identify changes to your code in Cloud Storage and trigger a retraining job.
B. Use the gcloud command-line tool to submit training jobs on AI Platform when you update your code.
C. Use Cloud Build linked with Cloud Source Repositories to trigger retraining when new code is pushed to the repository.
D. Create an automated workflow in Cloud Composer that runs daily and looks for changes in code in Cloud Storage using a sensor.
Correct Answer: C
Explanation:
When developing ML models, especially in an environment where frequent code updates and retraining are required (like in your case with image segmentation on CT scans), it's important to have an automated, cost-effective, and version-controlled process that minimizes manual intervention.
Here’s why C is the best choice:
Cloud Build is a fully managed continuous integration service in Google Cloud, which can automatically trigger a build or job when new code is pushed to a Cloud Source Repository or GitHub repository.
The process is as follows:
Cloud Build can automatically run a retraining job on AI Platform whenever a code update is pushed to the repository.
This solution is fully integrated into Google Cloud, so you get version control for your code and seamless integration with the rest of the GCP services.
Minimizing manual intervention is achieved because you set up automatic triggers for retraining, and it ensures computation efficiency by only triggering retraining jobs when necessary (e.g., after code changes).
Using Cloud Build ensures your ML models are retrained automatically with the latest code without needing additional manual steps, optimizing for both cost and performance.
A. Use Cloud Functions to identify changes to your code in Cloud Storage and trigger a retraining job
While Cloud Functions can respond to changes in Cloud Storage, using it for model retraining is not ideal. Cloud Functions are better suited for lightweight tasks, and using them to trigger training jobs can add unnecessary complexity and latency. Furthermore, this solution lacks version control for your code, which is critical when iterating on ML models.
B. Use the gcloud command-line tool to submit training jobs on AI Platform when you update your code
Using gcloud in a manual process is error-prone and requires consistent manual intervention. Every time you update your code, you would need to execute the command to submit training jobs. This is not automated and leads to inefficiencies, particularly when dealing with frequent code changes.
D. Create an automated workflow in Cloud Composer that runs daily and looks for changes in code in Cloud Storage using a sensor
While Cloud Composer is excellent for orchestrating complex workflows, it is overkill for this particular scenario. It would add unnecessary overhead by checking for code changes daily when a simpler Cloud Build trigger based on code push would be more efficient. Cloud Composer also introduces more complexity in terms of maintenance and setup, making it less suitable for this use case.
C is the optimal choice. It integrates version control with Cloud Build and Cloud Source Repositories, triggers retraining jobs automatically upon code updates, and eliminates the need for manual intervention, while ensuring efficient and cost-effective training operations. This solution balances automation, version control, and cost optimization, which are all critical factors when iterating on ML models.
Question No 10:
Your team needs to build a model that predicts whether images contain a driver's license, passport, or credit card. The data engineering team already built the pipeline and generated a dataset composed of 10,000 images with driver's licenses, 1,000 images with passports, and 1,000 images with credit cards. You now have to train a model with the following label map: [drivers_license', passport', `credit_card'].
Which loss function should you use?
A. Categorical hinge
B. Binary cross-entropy
C. Categorical cross-entropy
D. Sparse categorical cross-entropy
Answer: C
Explanation:
To determine the correct loss function, it’s important to first understand the problem and its requirements. You’re dealing with a multiclass classification task where the model must classify images into one of three categories: driver's license, passport, or credit card. Let's review the options in the context of this scenario:
Categorical hinge loss is commonly used with support vector machines (SVMs) for multiclass classification problems. This loss function is not typically used in neural networks for multiclass classification, especially when the output is a probability distribution over classes, as is common in tasks like this one. It’s more common in problems where the goal is to find the maximum margin between classes (such as in SVMs), and hence it’s not the best choice for this deep learning task.
Binary cross-entropy is used for binary classification tasks, where the model outputs probabilities for two classes (e.g., class 0 and class 1). Since you have three possible classes (driver's license, passport, credit card), binary cross-entropy is not suitable. This function would require you to set up multiple binary classifiers (one per class), which isn't ideal for this multiclass problem.
Categorical cross-entropy is the most commonly used loss function for multiclass classification problems where each instance belongs to one of several classes (in this case, three possible classes: drivers_license, passport, or credit_card). This loss function assumes the model outputs a probability distribution over the classes (using a softmax activation function in the output layer) and calculates the loss based on how far off the predicted probabilities are from the true class labels. Since your task involves predicting one of three categories, this is the correct choice.
Sparse categorical cross-entropy is similar to categorical cross-entropy, but it is used when the target labels are integers rather than one-hot encoded vectors. If your dataset contains labels like 0, 1, or 2 instead of ["drivers_license", "passport", "credit_card"], then sparse categorical cross-entropy would be the correct choice. However, since the labels are in string format (not integer-encoded), you would need to either manually convert the labels into integers or use categorical cross-entropy instead.
Since the model is a multiclass classification problem and the labels are in string format, the correct loss function to use is categorical cross-entropy. This loss function works with one-hot encoded labels or label indices that are converted internally into the appropriate one-hot format, which matches your current dataset structure.
Thus, the correct answer is C.
Top Training Courses
LIMITED OFFER: GET 30% Discount
This is ONE TIME OFFER
A confirmation link will be sent to this email address to verify your login. *We value your privacy. We will not rent or sell your email address.
Download Free Demo of VCE Exam Simulator
Experience Avanset VCE Exam Simulator for yourself.
Simply submit your e-mail address below to get started with our interactive software demo of your free trial.