Use VCE Exam Simulator to open VCE files
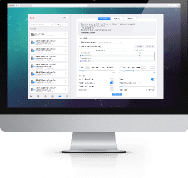
100% Latest & Updated Microsoft Azure AI AI-102 Practice Test Questions, Exam Dumps & Verified Answers!
30 Days Free Updates, Instant Download!
AI-102 Premium Bundle
Microsoft AI-102 Practice Test Questions, Microsoft AI-102 Exam Dumps
With Examsnap's complete exam preparation package covering the Microsoft AI-102 Practice Test Questions and answers, study guide, and video training course are included in the premium bundle. Microsoft AI-102 Exam Dumps and Practice Test Questions come in the VCE format to provide you with an exam testing environment and boosts your confidence Read More.
ExamSnap's Microsoft AI-102 Practice Test Questions and Exam Dumps, study guide, and video training course are complicated in premium bundle. The Exam Updated are monitored by Industry Leading IT Trainers with over 15 years of experience, Microsoft AI-102 Exam Dumps and Practice Test Questions cover all the Exam Objectives to make sure you pass your exam easily.
Purchase Individually
AI-102 Training Course
LIMITED OFFER: GET 30% Discount
This is ONE TIME OFFER
A confirmation link will be sent to this email address to verify your login. *We value your privacy. We will not rent or sell your email address.
Download Free Demo of VCE Exam Simulator
Experience Avanset VCE Exam Simulator for yourself.
Simply submit your e-mail address below to get started with our interactive software demo of your free trial.