Use VCE Exam Simulator to open VCE files
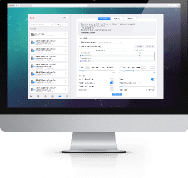
100% Latest & Updated Microsoft Azure AI AI-900 Practice Test Questions, Exam Dumps & Verified Answers!
30 Days Free Updates, Instant Download!
AI-900 Premium Bundle
Download Free AI-900 Exam Questions
File Name | Size | Download | Votes | |
---|---|---|---|---|
File Name microsoft.examlabs.ai-900.v2024-08-26.by.bonnie.78q.vce |
Size 755.69 KB |
Download 173 |
Votes 1 |
|
File Name microsoft.pass4sures.ai-900.v2022-01-29.by.max.74q.vce |
Size 793.88 KB |
Download 1036 |
Votes 1 |
|
File Name microsoft.pass4sures.ai-900.v2021-10-27.by.grace.75q.vce |
Size 752.46 KB |
Download 1130 |
Votes 1 |
|
File Name microsoft.examcollection.ai-900.v2021-09-24.by.layla.66q.vce |
Size 811.06 KB |
Download 1163 |
Votes 1 |
|
File Name microsoft.prep4sure.ai-900.v2021-09-06.by.mila.53q.vce |
Size 896.22 KB |
Download 1179 |
Votes 1 |
|
File Name microsoft.pass4sure.ai-900.v2021-05-15.by.levi.54q.vce |
Size 791.93 KB |
Download 1298 |
Votes 1 |
|
File Name microsoft.selftestengine.ai-900.v2021-02-12.by.bobby.51q.vce |
Size 786.62 KB |
Download 1410 |
Votes 2 |
Microsoft AI-900 Practice Test Questions, Microsoft AI-900 Exam Dumps
With Examsnap's complete exam preparation package covering the Microsoft AI-900 Practice Test Questions and answers, study guide, and video training course are included in the premium bundle. Microsoft AI-900 Exam Dumps and Practice Test Questions come in the VCE format to provide you with an exam testing environment and boosts your confidence Read More.
The field of artificial intelligence (AI) has rapidly evolved, becoming an integral part of various industries and technologies. Recognizing the significance of AI, Microsoft offers the AI-900: Microsoft Azure AI Fundamentals exam, providing individuals with an opportunity to showcase their understanding of machine learning and AI principles within the context of Microsoft Azure services.
The AI-900 exam leads to the Microsoft Certified: Azure AI Fundamentals certification. This certification is designed for individuals possessing diverse backgrounds encompassing both technical and non-technical expertise, making it accessible to a wide range of professionals. Although having experience in data science and software engineering is not obligatory, a basic understanding of cloud concepts and applications based on a client-server architecture.
The Microsoft AI-900 certification exam typically consists of 40-60 questions, and candidates have a duration of 45 minutes to complete the assessment. The exam fee is $99, making it a cost-effective way to validate one's understanding of AI concepts on the Azure platform.
Exam AI-900 encompasses five main topics, each contributing to a well-rounded understanding of AI workloads on Azure:
- Describe Artificial Intelligence workloads and considerations (15–20%): This section focuses on providing an understanding of different AI workloads and the considerations involved in their implementation.
- Describe fundamental principles of machine learning on Azure (20–25%): Candidates are expected to demonstrate knowledge of the basic principles that underpin machine learning and how they are implemented on the Azure platform.
- Describe features of computer vision workloads on Azure (15–20%): This topic explores the capabilities and functionalities of computer vision workloads, emphasizing their application within the Azure environment.
- Describe features of Natural Language Processing (NLP) workloads on Azure (15–20%): Candidates are required to understand the features and applications of Natural Language Processing (NLP) workloads, specifically within the Azure ecosystem.
- Describe features of generative AI workloads on Azure (15–20%): This section delves into the features and considerations related to generative AI workloads, showcasing their relevance and applicability on the Azure platform.
Successful completion of the AI-900 exam leads to the Microsoft Certified: Azure AI Fundamentals certification. This certification serves as a foundational stepping stone for individuals looking to delve deeper into AI and machine learning on the Azure platform. It is particularly beneficial for those aspiring to pursue more advanced Azure role-based certifications, such as Azure Data Scientist Associate or Azure AI Engineer Associate.
Earning the Microsoft Certified: Azure AI Fundamentals certification through the AI-900 exam comes with a multitude of benefits, making it a valuable investment in one's professional development. This certification not only serves as a testament to your foundational knowledge in artificial intelligence but also opens up a range of opportunities across diverse industries.
Here are several compelling benefits of successfully passing the Microsoft AI-900 exam:
- Career Jump-Start: Mastering the basics in AI through the AI-900 exam can provide individuals with the necessary skills to jump-start their career in the rapidly evolving field of artificial intelligence.
- Gateway to Technical Opportunities: The certification serves as a gateway to various technical opportunities offered by Azure. It equips individuals with the knowledge needed to explore and excel in diverse areas, including healthcare, financial management, and environmental protection.
- Solution Building: After passing the AI-900 certification exam, individuals can build innovative solutions and enhance their applications by leveraging AI capabilities. The certification gained empowers professionals to contribute to technological advancements in multiple industries.
- Demonstrate Knowledge: The Microsoft Certified: Azure AI Fundamentals certification obtained after successful completion of the AI-900 exam validates foundational knowledge of machine learning and AI concepts, along with a deep understanding of how these concepts are applied using Azure services. This recognition is valuable for individuals seeking to showcase their expertise to potential employers and peers.
In conclusion, the AI-900: Microsoft Azure AI Fundamentals exam is a crucial step for individuals aspiring to establish their expertise in AI and machine learning on the Azure platform. With a focus on foundational principles and hands-on knowledge, this exam opens doors to a world of possibilities, allowing professionals to contribute to advancements in various industries. The Microsoft Certified: Azure AI Fundamentals certification not only proves one's skills in AI but also provides a solid foundation for pursuing more advanced certifications, making it a valuable asset for anyone looking to thrive in the dynamic field of artificial intelligence.
Opting for the AI-900 video course in your preparation process is essential for several reasons. Firstly, video courses provide a dynamic and engaging learning experience, leveraging visual and auditory elements to enhance comprehension. The complex topics covered in the AI-900 exam, such as machine learning principles and Azure services, are effectively explained through real-world examples and demonstrations in video format. Additionally, video courses offer flexibility, allowing learners to pace themselves and revisit challenging concepts. The AI-900 video course ensures a comprehensive understanding of the exam domains, making it a valuable resource for candidates aiming to excel in the Microsoft Certified: Azure AI Fundamentals certification.
The first domain of the AI-900 exam, "Artificial Intelligence workloads and considerations," delves into the practical applications and ethical dimensions of AI. Comprising 15-20% of the exam, this section evaluates candidates' ability to identify features of common AI workloads. From observing data and identifying anomalies to content moderation, computer vision, natural language processing, knowledge mining, and generative AI, candidates must recognize characteristics of generative AI solutions. Moreover, the domain assesses their understanding of the principles that guide the responsible development and implementation of AI, encompassing considerations such as fairness, reliability, safety, privacy, security, inclusiveness, transparency, and accountability. Successful mastery of this domain demonstrates a well-rounded grasp of AI applications and the ethical frameworks essential for responsible AI development and deployment.
The second domain of the AI-900 exam, "Fundamental principles of machine learning on Azure," accounts for 20–25% of the test and evaluates candidates on their comprehension of key machine learning principles and Azure's machine learning capabilities. Candidates are expected to identify common machine learning techniques, such as regression, classification, clustering, and deep learning scenarios. Fundamental concepts in machine learning include recognizing features and attributes and identifiers within datasets and understanding the application of datasets used for training and validation purposes. Additionally, candidates need to explain the capabilities of Azure Machine Learning, covering machine learning that is automated, model deployment and management features within the Azure Machine Learning framework, compute and data services for data science. Proficiency in this domain demonstrates a solid understanding of machine learning fundamentals and the ability to utilize Azure services for effective machine learning solutions.
The third domain of the AI-900 exam, "Features of computer vision workloads on Azure," constitutes 15–20% of the exam and evaluates candidates on their understanding of computer vision concepts and Azure's capabilities in this domain. Candidates are assessed on their ability to identify common types of computer vision solutions, including image classification, object detection, optical character recognition, facial detection, and facial analysis. Furthermore, candidates must be familiar with Azure services and tools for tasks related to computer vision. This involves describing the features and functionalities provided by the Azure AI Vision service, Azure AI Face detection service, and Azure AI Video Indexer service. Proficiency in this domain showcases the candidate's ability to comprehend and apply computer vision features and leverage Azure services for effective implementation in various visual recognition tasks.
The fourth domain of the AI-900 exam, "Features of Natural Language Processing (NLP) workloads on Azure," represents 15–20% of the test and evaluates candidates on their understanding of NLP concepts and proficiency in leveraging Azure services for language-related tasks. Candidates are assessed on their ability to identify features and applications of typical scenarios involving Natural Language Processing workloads, including identifying and extracting key phrases, entity recognition, sentiment analysis, language modeling, recognition and generation of speech, and translation. Additionally, candidates must be familiar with Azure services and tools for tasks related to NLP, such as the Azure AI Language service, Azure AI Speech service, and Azure AI Translator service. Proficiency in this domain demonstrates a candidate's capability to work with diverse NLP tasks using Azure's advanced language processing services, highlighting their competence in natural language understanding and processing within the Azure ecosystem.
The fifth domain of the AI-900 exam, "Features of generative AI workloads on Azure," constitutes 15–20% of the test and evaluates candidates on their understanding of generative AI concepts and proficiency in utilizing Azure services for generative tasks. Candidates are expected to identify features of generative AI solutions, including recognizing characteristics of generative AI models and understanding common scenarios for their application. Additionally, candidates must exhibit awareness of responsible AI considerations specific to generative AI. The domain further assesses candidates on their knowledge of the capabilities of the Azure OpenAI Service, encompassing natural language generation, code generation, and image generation. Proficiency in this domain signifies a candidate's ability to harness generative AI technologies within the Azure ecosystem, demonstrating competence in creating content and generating solutions across various domains.
Microsoft AI-900 exam dumps and practice test questions play a crucial role in effective exam preparation, and ExamSnap is a valuable resource for accessing these materials. The AI-900 exam assesses foundational knowledge of Azure AI services, making it essential to thoroughly prepare. Exam dumps on ExamSnap provide candidates with a real exam environment, allowing them to familiarize themselves with the question format and evaluate their readiness. Practice test questions offered by ExamSnap are designed to cover the exam objectives comprehensively, enabling candidates to identify areas of improvement and strengthen their knowledge. These resources are vital for enhancing confidence and ensuring success on the AI-900 exam. By leveraging ExamSnap's platform, aspiring Microsoft Certified: Azure AI Fundamentals professionals can enhance their preparation and increase their chances of achieving a successful outcome.
ExamSnap's Microsoft AI-900 Practice Test Questions and Exam Dumps, study guide, and video training course are complicated in premium bundle. The Exam Updated are monitored by Industry Leading IT Trainers with over 15 years of experience, Microsoft AI-900 Exam Dumps and Practice Test Questions cover all the Exam Objectives to make sure you pass your exam easily.
Comments (0)
Please post your comments about Microsoft Exams. Don't share your email address asking for AI-900 braindumps or AI-900 exam pdf files.
Purchase Individually
AI-900 Training Course
Latest IT Certification News
LIMITED OFFER: GET 30% Discount
This is ONE TIME OFFER
A confirmation link will be sent to this email address to verify your login. *We value your privacy. We will not rent or sell your email address.
Download Free Demo of VCE Exam Simulator
Experience Avanset VCE Exam Simulator for yourself.
Simply submit your e-mail address below to get started with our interactive software demo of your free trial.