Use VCE Exam Simulator to open VCE files
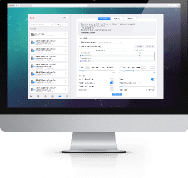
Get 100% Latest Microsoft Certified: Azure Data Scientist Associate Practice Tests Questions, Accurate & Verified Answers!
30 Days Free Updates, Instant Download!
DP-100 Premium Bundle
Microsoft Certified: Azure Data Scientist Associate Certification Practice Test Questions, Microsoft Certified: Azure Data Scientist Associate Exam Dumps
ExamSnap provides Microsoft Certified: Azure Data Scientist Associate Certification Practice Test Questions and Answers, Video Training Course, Study Guide and 100% Latest Exam Dumps to help you Pass. The Microsoft Certified: Azure Data Scientist Associate Certification Exam Dumps & Practice Test Questions in the VCE format are verified by IT Trainers who have more than 15 year experience in their field. Additional materials include study guide and video training course designed by the ExamSnap experts. So if you want trusted Microsoft Certified: Azure Data Scientist Associate Exam Dumps & Practice Test Questions, then you have come to the right place Read More.
Study with ExamSnap to prepare for Microsoft Certified: Azure Data Scientist Associate Practice Test Questions and Answers, Study Guide, and a comprehensive Video Training Course. Powered by the popular VCE format, Microsoft Certified: Azure Data Scientist Associate Certification Exam Dumps compiled by the industry experts to make sure that you get verified answers. Our Product team ensures that our exams provide Microsoft Certified: Azure Data Scientist Associate Practice Test Questions & Exam Dumps that are up-to-date.
Microsoft Training Courses
LIMITED OFFER: GET 30% Discount
This is ONE TIME OFFER
A confirmation link will be sent to this email address to verify your login. *We value your privacy. We will not rent or sell your email address.
Download Free Demo of VCE Exam Simulator
Experience Avanset VCE Exam Simulator for yourself.
Simply submit your e-mail address below to get started with our interactive software demo of your free trial.